Bringing the digital underground
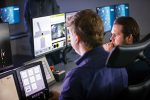
Monitoring autonomous and digital mining operations from Epiroc’s control room in Sweden. CREDIT: EPIROC
With respect to implementing a digital platform in an underground mine, justification can seem straightforward, including:
- Enhanced communication using cell phone or VOIP phone;
- Ability to track asset and personnel position in real time; and
- Telemetric data from equipment, including engine hours, alarm conditions, production related metrics.
That being said, cost and associated payback can vary widely and depends upon the needs of the operation and how the data generated will be used. Pending the maturity of the operation on the technology curve and ability to use the data generated, all that may be needed for an immature site is basic communication (radio via leaky feeder) and, say, the ability to find equipment and people relatively easily. That can be done inexpensively. Other operations that are larger in scope and that have a sound technology infrastructure and personnel to use the data, can make use of a more sophisticated digital roll-out, including:
- Wi-Fi or LTE infrastructure;
- Asset and personnel positioning in real time. For example, Mobilaris Mining Intelligence (MMI);
- Electronic scheduling to monitor planned vs. actual performance and correction to schedule. For example, Deswick Operations;
- Cellphone and related app usage;
- Equipment telemetrics (equipment related information plus production related data). For example, the Certiq platform from Epiroc; and
- Tablet usage for Short Interval Control (SIC) related activities.
Taken at surface value, justification for a digital platform can seem selfevident; better communication, automatic data generation, real-time positioning of assets and people, etc. However, sometimes not enough thought is given to what data is actually required and how it will be used. As such, excessive cost can be incurred for various products for reduced payback if a solid business case is not developed first.
In many cases, mines collect all kinds of data, ranging from equipment reliability information to production data, but analyzing the data can be a low priority. In many cases, resources are not assigned or dedicated to look at this data. At best, only bits of the data collected is used to do a retrospective analysis in case of an incident, for historical analysis on occasion for deviation in production, or finding equipment and personnel. Data is most powerful when we use it to analyze the past and make predictions for future performance. Predictions of future performance act as a guide when it comes to operational planning. For example, data gleaned from an intelligent production drill can tell us that if we drill for X amount of hours, we can expect Y metres. From this perspective, the problem can be reframed: not how do we gain Y metres, but how do we make the process more efficient to gain more hours. We cannot control metres, but we can control hours.
As noted above, we can put in a digital platform and associated communications infrastructure, and not use it to its full potential. Therefore, return on investment is not optimal. As such, it is important to plan for the types of data that can be accessed and how it can be used for improvement, as this will improve return on investment.
Return on investment for a digital infrastructure can be done on a pilot scale, just like other new investments, to prove its worth and gain learnings for a larger roll-out. Pending upon scale and products chosen, data generated from the pilot can be used to justify a larger roll-out – i.e. develop the business case. Developing a business case for digitalization can be a straightforward process to show how the data generated can be used to make an improvement.
In order to make the best use of data collected from a pilot digitalization roll-out, we need to have an improvement process in place: the scientific method:
- Ask a question;
- Do research;
- Make a hypothesis;
- Conduct an experiment;
- Analyze the data; and • Accept or reject the hypothesis.
This method is independent of how we collect data, whether by hand or by a digital infrastructure. However, combining both methods is the best as one method validates the other method, and tells you if the information collected passes the “smell test” (i.e. information makes sense as I have personally observed and worked with it).
The following is an example of utilizing the scientific method to make an improvement, and subsequently support the business case for digitalization:
1 Ask a Question. If I increase my average scoop engine hours per shift from 7.5 hours to 8.5 hours, can I correspondingly increase my buckets per shift by the same percentage?
2 Do Research. Collect historical data to check for correlation between scoop engine hours and buckets. Measure current mucking performance through manual data collection, in combiit make sense? Do they tell similar stories? Determine how much bucket count is influenced by engine hours. Understand and validate what can be physically improved/changed to improve bucket count.
3 Make a Hypothesis. Your hypothesis could be: I can increase scoop engine hours by reducing time to find equipment, fueling, and reducing congestion on the ramp. This will be done via monitoring equipment in real time (i.e. digital platform) such that activities that negatively impact mucking are readily addressed (e.g. finding equipment, making fueling and scoop maintenance a priority, keeping ramps free for truck traffic).
4 Conduct an Experiment. Install an underground communications infrastructure (Wi-Fi of LTE) with a digital management system (e.g. MMI) to readily collect equipment position and telemetry data. This can be done on a pilot scale to justify the installation of a larger roll-out. Develop standards for mucking to gain targeted mucking hours – have mucking operations run to these standards. Develop a management structure (control and response plan) to execute the experiment.
5 Analyze the Data. Use data from the digital platform (plus manual data collection) and associated analysis to validate the hypothesis: Did I increase average scoop engine hours from 7.5 to 8.5 hours, and if so, did I increase associated bucket count? Look for effects (both anticipated and unanticipated) where digitalization affected operations. Use graphical and statistical data as well as interviews with stakeholders in the experiment to quantify.
6 Accept or Reject the Hypothesis. If in fact a desirable outcome was achieved, how large was the improvement – was it cost effective? Does it justify a larger scale roll-out of the digital platform? What are the related benefits of having a digital platform that may not be directly tied to improved performance?
As shown in the above example, we can quantify the return of installing a digital platform. Use of the scientific method in combination with the data a digital platform provides gives us an improvement methodology to improve operations – either through small, rapid improvements, or larger, more complex improvements. The key is to measure first to understand your problem, implement the desired solution, and measure again to verify impact. Related improvement methodologies like Lean Manufacturing or 6Sigma applied to the underground can have a more significant impact when underpinned by a digital infrastructure.
Ken Schroeder is digitalization project manager with Epiroc.
Comments